Summer School Lectures: GAN methods for Medical Imaging
This lecture explains deep generative models in medical imaging, covering GANs, VAEs, diffusion models, and real-world medical applications.
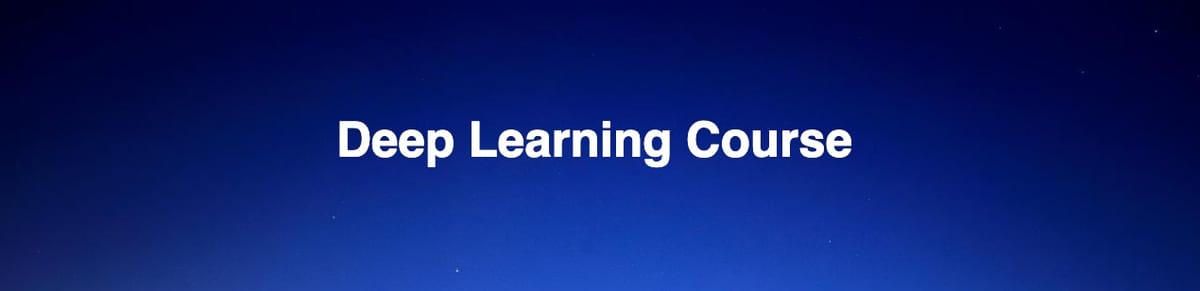
Hi Welcome back again, here is the third part of the summer school. In this lecture, Prof. Mohammadhadi Shateri explains the concept of deep generative models (DGMs) with a focus on their application in medical imaging. He begins by introducing generative models, which aim to simulate the underlying data distribution and generate new samples, distinguishing them from discriminative models that focus on classification tasks. Prof. Shateri then dives into various types of DGMs like GANs, VAEs, autoregressive models, and diffusion models, explaining their architectures, training methods, and use cases. He covers real-world applications of DGMs in medical imaging, such as data augmentation, anomaly detection, image translation (e.g., MRI to CT), and image enhancement. He also highlights evaluation metrics such as SSIM, PSNR, and FID for assessing the quality of generated medical images, using case studies to illustrate how DGMs improve tasks like segmentation, denoising, and translation in medical diagnostics.
Enjoy the lecture:
Part 1: Introduction
Part 2: Theory of Deep Generative models: GAN
Part 2: Theory of Deep Generative models: Diffusion Models
Part 3: Deep Generative models in Medical Imaging (Case Studies)
I hope you enjoyed the lecture. If you did, please consider sharing and subscribing—it helps keep me motivated!
See you soon 🤗